Estimating the total number of phosphoproteins and phosphorylation sites in eukaryotic proteomes.
Download Supplementary Files of this Paper
Phosphorylation is the most frequent post-translational modification made to proteins and may regulate protein activity as either a molecular digital switch or a rheostat. Despite the cornucopia of high-throughput (HTP) phosphoproteomic data in the last decade, it remains unclear how many proteins are phosphorylated and how many phosphorylation sites (p-sites) can exist in total within a eukaryotic proteome.
At present, values for these numbers remain in the realm of speculation. It has been suggested that the biological activity of between 1/3 and 2/3 of an organism’s proteome could be regulated by post-translational phosphorylation [1–4]. In the specific case of the human proteome, it has been proposed that 57,000, 500,000, 700,000, or even 1,000,000 p-sites may exist [5–8]. A deep phosphoproteome analysis on HeLa cells estimated that at least 75% of the proteome expressed in those cells can be phosphorylated, and this number may well rise to 90%, if phosphoproteomic experiments are performed at higher coverage [9].
In an effort to provide a reasonable and statistically defensible estimate based on current knowledge, we have mined over 1000 articles from the literature and gathered and filtered 187 publicly available HTP phosphoproteomic datasets from four well-studied species. By implementing two independent statistical methods - the Capture-Recapture method, and Curve-Fitting on the saturation curve of redundant phosphoproteins/p-sites vs non-redundant phosphoproteins/p-sites - we have obtained, for the first time, a reliable estimate of their total number for humans and three other model eukaryotes (mouse, Arabidopsis, and yeast).
Estimates were also adjusted for different levels of noise within the individual datasets and other confounding factors. We estimate that in total, 13,000, 11,000 and 3,000 phosphoproteins and 230,000, 156,000 and 40,000 p-sites exist in human, mouse and yeast, respectively, whereas estimates for Arabidopsis were not as reliable.
Conclusions: Most of the phosphoproteins have been discovered for human, mouse and yeast, while the dataset for Arabidopsis is still far from complete. The datasets for p-sites are not as close to saturation as those for phosphoproteins, Integration of the LTP data suggests that current HTP phosphoproteomics appears to be capable of capturing 70-95% of total phosphoproteins, but only 40-60% of total p-sites.
Supplementary data contain the phosphoproteomic datasets in the four species (Suppl. Files S1-S4), the list of publications used for each species (Suppl. Excel File S5), a Quicktime screencasting video that shows how curve-fitting was performed in Microsoft Excel (Suppl. File S6) and an excel file that contains the Jaccard Distances between the various datasets for each species (Suppl. File S7). The supplementary excel file S8 contains the evaluation of the Capture-Recapture and Curve-fitting on the five yeast proteomics experiments (five proteases) (each randomly downsampled at 50%) of Sawney et al., 2010.
Human |
Mouse |
Arabidopsis |
Yeast |
||
---|---|---|---|---|---|
PROTEINS |
current |
10456 |
6512 |
4930 |
2587 |
current_3X |
6683 |
3827 |
1815 |
1630 |
|
Rcapture_HTP_vs_LTP |
12844 |
11190 |
NA |
2951 |
|
Rcapture_1%_noise |
10239 |
8346 |
6531 |
2772 |
|
CF_1%_noise |
9160 |
7213 |
4292 |
2373 |
|
CF_3X |
7582 |
6789 |
NA |
2297 |
|
CF_best_start_1%_noise |
8803 |
7167 |
4558 |
2328 |
|
CF_best_end_1%_noise |
8775 |
7099 |
4292 |
2304 |
|
CF_half_exp_1%_noise |
7885 |
6329 |
2373 |
2257 |
|
P-SITES |
current |
86181 |
36438 |
14796 |
13244 |
current_3X |
27110 |
10384 |
3078 |
4156 |
|
Rcapture_HTP_vs_LTP |
229616 |
155668 |
NA |
40350 |
|
Rcapture_1%_noise |
124985 |
71456 |
27815 |
21343 |
|
CF_1%_noise |
94670 |
54031 |
23531 |
14533 |
|
CF_3X |
91500 |
NA |
34457 |
NA |
|
CF_best_start_1%_noise |
82092 |
45797 |
15122 |
12962 |
|
CF_best_end_1%_noise |
86723 |
49122 |
23531 |
14496 |
|
CF_half_exp_1%_noise |
89639 |
36615 |
6016 |
11980 |
|
Table 1. Second column denotes the analysis and datasets: current: experimentally identified; current_3X: experimentally identified in three or more experiments; Rcapture_HTP_vs_LTP: The Capture-Recapture analysis that used the HTP compendium and the LTP compendium (shown in bold as the most reliable estimate); Rcapture_1%_noise: The Capture-Recapture analysis assuming 1% noise in each dataset; CF_1%_noise: The Curve-Fitting analysis assuming 1% noise; CF_3X: The Curve-Fitting analysis based on the datasets that have been identified in three or more experiments. CF_best_start_1%_noise: The Curve-Fitting analysis assuming 1% noise and changing the order of the largest experiment as first; CF_best_end_1%_noise: The Curve-Fitting analysis assuming 1% noise and changing the order of the largest experiment as last; CF_half_exp_1%_noise: The Curve-Fitting analysis assuming 1% noise and using only the first half of experiments. |
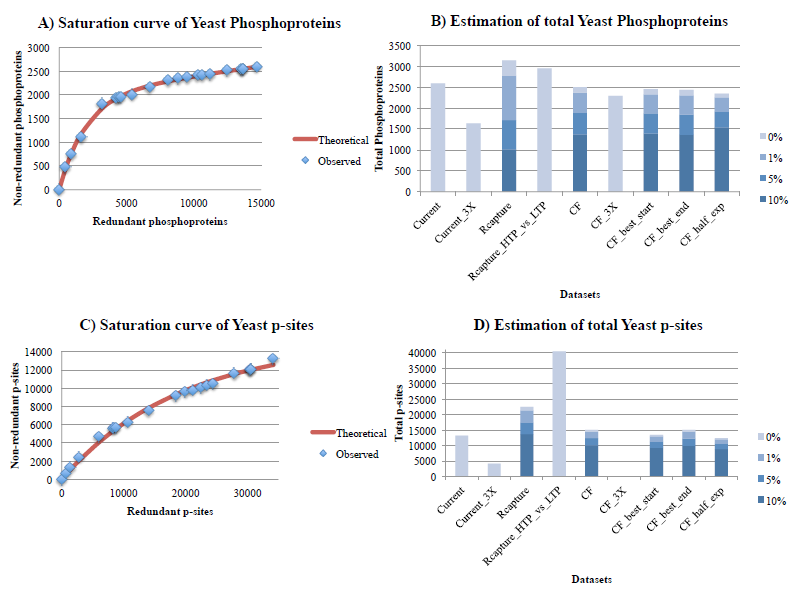
Figure 1. Estimation of the total number of phosphoproteins (1A, 1B) and p-sites (1C, 1D) for yeast, with the curve-fitting (assuming 1% noise) and Capture-Recapture methods, also correcting for 3 levels of noise (1%, 5%, 10%). In figures 1A and 1C, the x-axis is the cumulative number of redundant phosphoproteins/p-sites, whereas the y-axis is the cumulative number of non-redundant phosphoproteins/p-sites. The red curve is fitted for 1% noise. In figures 1B and 1D: Current is the total number of phosphoproteins/p-sites detected so far (by applying our filtering criteria). Current_3X is the total number of phosphoproteins/p-sites detected so far in at least 3 experiments. Rcapture is the estimation of maximum number of phosphoproteins/p-sites based on the Rcapture method (using the 15 largest datasets). Rcapture_HTP_vs_LTP is the estimation of maximum number of phosphoproteins/p-sites based on the Rcapture method, but this time using only two datasets, where one of them is the compendium of all HTP experiments and the second is the compendium of all LTP experiments from PhosphoGrid2. CF is the estimation of maximum number of phosphoproteins/p-sites based on the curve-fitting method of the saturation curve from all experiments. CF_3X is the estimation of maximum number of phosphoproteins/p-sites identified in at least 3 experiments, based on the curve-fitting method (in this case, a reasonable estimate was not possible). CF_best_start is the estimation of maximum number of phosphoproteins/p-sites based on the curve-fitting method of the saturation curve from all experiments, but this time, the largest experiment is used as first in the series. CF_best_end is the estimation of maximum number of phosphoproteins/p-sites based on the curve-fitting method of the saturation curve from all experiments, but this time, the largest experiment is used as last in the series. CF_half_exp is the estimation of maximum number of phosphoproteins/p-sites based on the curve-fitting method of the saturation curve from the first half experiments.
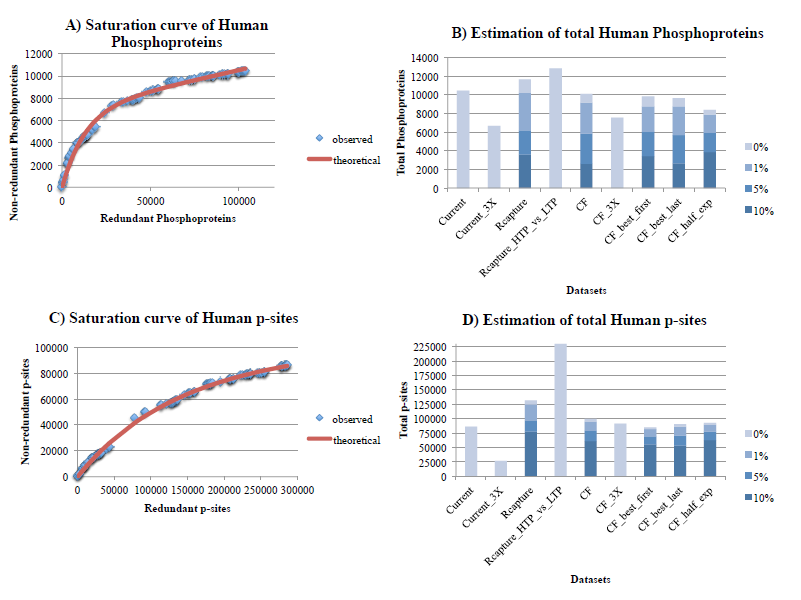
Figure 2. Estimation of the number of phosphoproteins (2A, 2B) and p-sites (2C, 2D) for human, with the Curve-Fitting (assuming 1% noise) and Capture-Recapture methods, also correcting for various levels of noise (1%, 5%, 10%). See legend of Figure 1 for explanations.
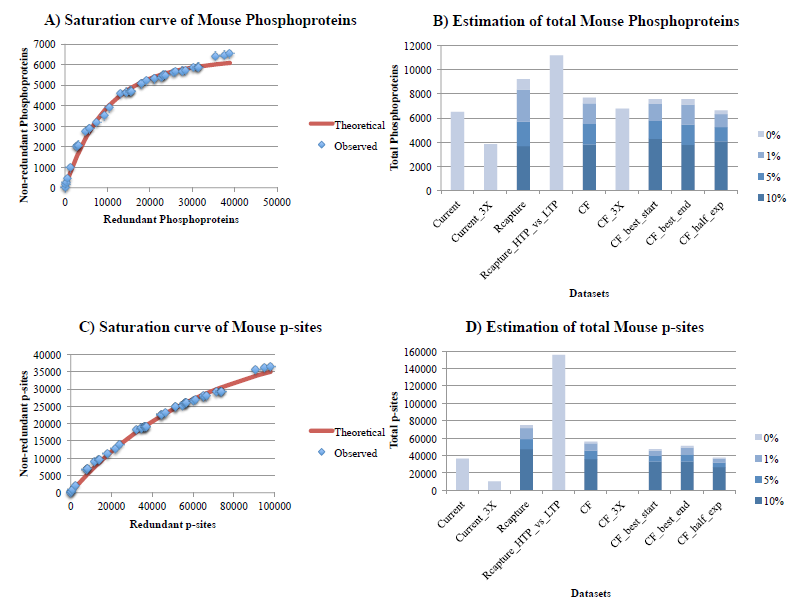
Figure 3. Estimation of the number of phosphoproteins (3A, 3B) and p-sites (3C, 3D) for mouse, with the Curve-Fitting (assuming 1% noise) and Capture-Recapture methods, also correcting for 3 levels of noise (1%, 5%, 10%). See legend of figure 1 for explanations. Estimates on figures 3B and 3D are obtained for a Vega annotated proteome of 16,000 protein-coding genes, where all estimates have been readjusted 25% upwards.
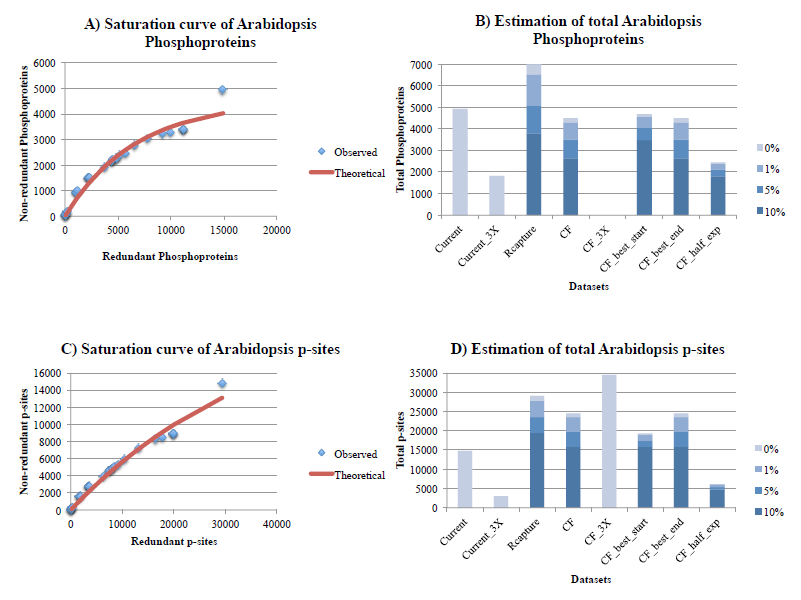
Figure 4. Estimation of the number of phosphoproteins (4A, 4B) and p-sites (4C, 4D) for Arabidopsis, with the Curve-Fitting (assuming 1% noise) and Capture-Recapture methods, also correcting for 3 levels of noise (1%, 5%, 10%). See legend of Figure 1 for explanations.
- Amoutzias GD, He Y, Lilley KS, Van de Peer Y, Oliver SG. Evaluation and properties of the budding yeast phosphoproteome. Mol. Cell Proteomics. 2012;11:M111.009555.
- Cohen P. The origins of protein phosphorylation. Nat. Cell Biol. 2002;4:E127-130.
- Pinna LA, Ruzzene M. How do protein kinases recognize their substrates? Biochim. Biophys. Acta. 1996;1314:191–225.
- Sadowski I, Breitkreutz B-J, Stark C, Su T-C, Dahabieh M, Raithatha S, et al. The PhosphoGRID Saccharomyces cerevisiae protein phosphorylation site database: version 2.0 update. Database (Oxford). 2013;2013:bat026.
- Boekhorst J, Boersema PJ, Tops BBJ, van Breukelen B, Heck AJR, Snel B. Evaluating experimental bias and completeness in comparative phosphoproteomics analysis. PLoS ONE. 2011;6:e23276.
- Boersema PJ, Foong LY, Ding VMY, Lemeer S, van Breukelen B, Philp R, et al. In-depth qualitative and quantitative profiling of tyrosine phosphorylation using a combination of phosphopeptide immunoaffinity purification and stable isotope dimethyl labeling. Mol. Cell Proteomics. 2010;9:84–99.
- Lemeer S, Heck AJR. The phosphoproteomics data explosion. Curr Opin Chem Biol. 2009;13:414–20.
- Ubersax JA, Ferrell JE. Mechanisms of specificity in protein phosphorylation. Nat. Rev. Mol. Cell Biol. 2007;8:530–41.
- Sharma K, D’Souza RCJ, Tyanova S, Schaab C, Wiśniewski JR, Cox J, et al. Ultradeep human phosphoproteome reveals a distinct regulatory nature of Tyr and Ser/Thr-based signaling. Cell Rep. 2014;8:1583–94.